In 2017, CEOs arrived at the conclusion that machine learning and artificial intelligence (ML / AI) will be critical to unlock competitive advantages in the future. However, most enterprises had very little understanding of exactly what is possible today and how much value the investment in various ML / AI technologies can bring. Here are my six key recommendations for 2018:
Recommendation #1: Always Question the Perceived Limits of Machine Learning
Often the perceived bottlenecks of ML / AI do not exist or are trivial. The actual implementation challenges often originate from ‘simple stuff’ such as missing data connectors, complex user interfaces, and, most importantly, a general lack of understanding of the specific capabilities of ML / AI.
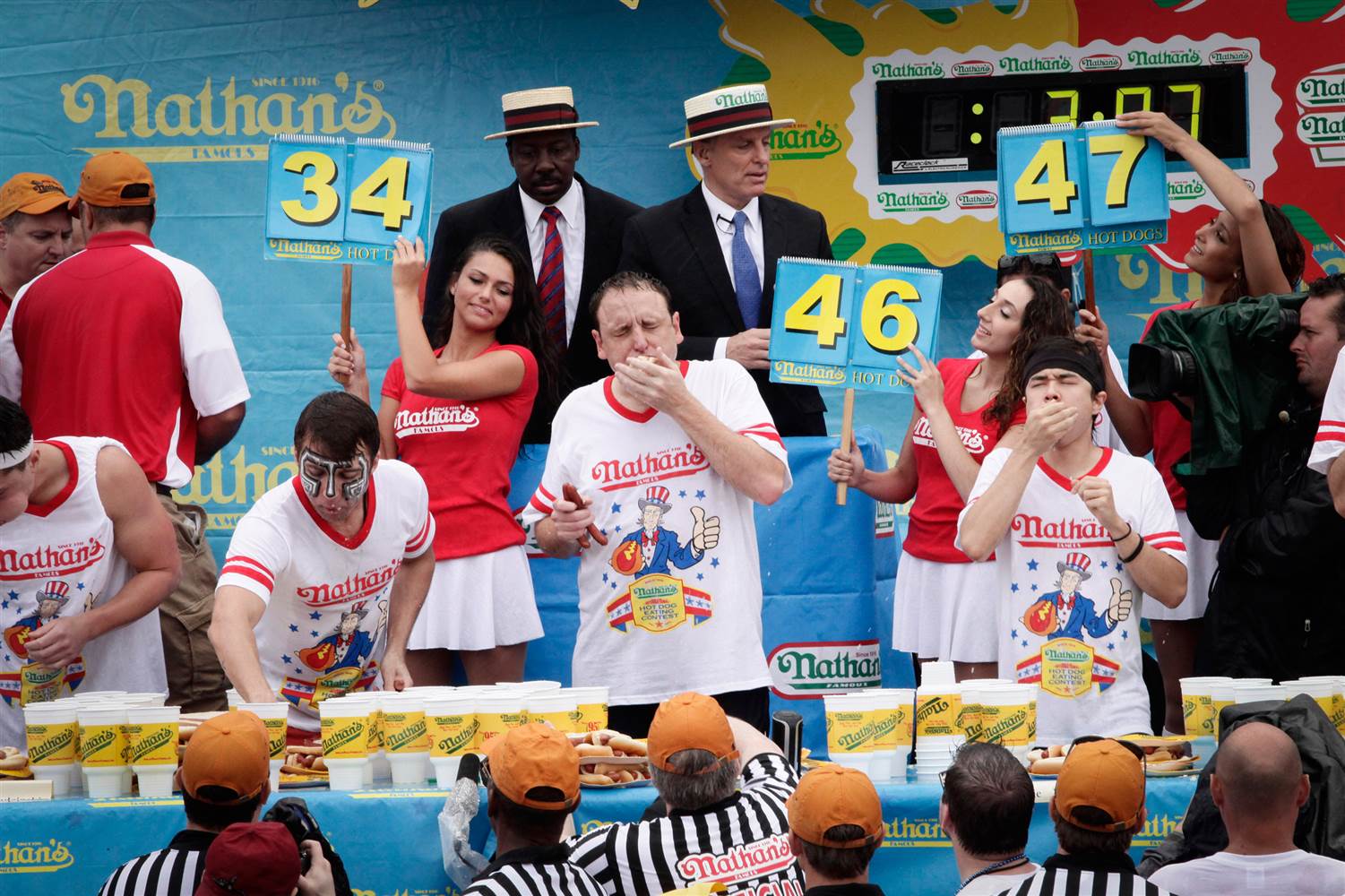
Examples: My favorite example is the “focused” inbox of MS Outlook 2016 that saves me tremendous time and prevents me from overlooking relevant emails on a daily basis. Another example is the “Google Explorer” that was added to Google Docs in mid 2017. While writing these recommendations, Google Explorer reminded me of relevant passages of my previous publications that I ended up considering and linking too. This saved me tremendous time and, in my opinion, improved the quality of this specific article. The same is true for more data-center directed examples where it may at first seem that ML / AI is not applicable, but in reality could for example dramatically reduce complexity for VMware or application administrators, saving them time and preventing mistakes.
Recommendation #2: Demand Clear Success Metrics for AI / ML Projects
AI / ML projects are not exempt from the basic principles of project management. Therefore, clear success metrics and milestones are critical not only to monitor project progress but also to manage expectations upfront. This is especially important to demystify ML / AI and create a joint understanding of what the short and long term advantages should be.
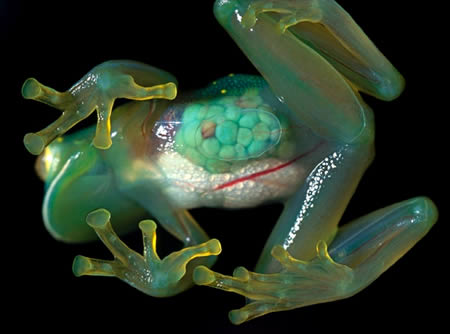
Example: Talking to customers about ML / AI over the last decade has been eye opening to me as it seems like everyone has a slightly different understanding of this topic. The first group of customers is regarding ML / AI as a ‘handy utility’ to improve the reliability of object recognition, processing bank transactions, or reducing the number of mistakes in medical prescriptions. Defining metrics for these types of projects is simple, as we only need to compare the errors made by ML / AI to the error rate from before.
Then there’s a much more ambitious group that would like to see ML / AI answering complex questions such as ‘should I lend this guy money, and how much?’, ‘does the risk of a certain investment outweigh the cost’, ‘should I first tend to a high severity database issue that only impacts a few users today, or should I focus my resources on fixing an unrelated issue that could cause much more wide-ranging problems in the future.’ Defining metrics for these projects is much more difficult as we often are unable to identify causalities between independent and dependent variables. This goes down to the ‘root challenge’ of all of ML / AI, where algorithms can only map a small number of very specific aspects of reality, as they do not have access to all relevant context data and b) as it is difficult to determine the effect of a specific decision.
Recommendation #3: Encourage Your Staff to ‘Think Further’
Remember when voice control in our cars was just a gimmick and Alexa or Cortana-like capabilities were considered Science Fiction? When I wrote earlier this year that the new vSphere should come with a built in AI / ML administrator I received a lot of questions and smiles about this ‘clearly exaggerated’ request. Interestingly, I also received feedback from ‘folks on the ground’ in terms of ‘DRS is basically like ML,’ or ‘here’s this other hypervisor feature that automates decisions x, y, and z.’ I absolutely do not want to belittle this feedback or these great hypervisor features, but let’s remember that all of this still has led enterprise computing into the arms of the megaclouds. And now exactly these megaclouds are ‘racing up the stack’ to entirely getting out of the way of the developers. Therefore, it is critical to allow your staff to define their environments outside of the boundaries of enterprise IT. This is critical to not lose sight of the long term vision of ML / AI: optimizing our decisions and automating responses, based on our exact priorities.
Example: ‘Where should we start with ML / AI,’ is a question we constantly get from the C-Level. The challenge is to find a healthy compromise between enabling staff to find impactful new business solutions that may not be entirely feasible today, while not distracting staff from their ‘day jobs.’ Similar to DevOps, ML / AI should not be isolated within a specific group or competence center, but needs to be pervasive across the organization, as the truly life changing value will come from business staff.
Recommendation #4: Understand the Tradeoffs between Deep Learning and Narrow Modelling
Deep learning is the ‘topic de jour’ as it has the aura of AI as we know it from the movies. In reality ‘deep learning’ helps the machine to independently determine which aspects of its environment are relevant in respect to a specific topic. However, for numerous reasons, the machine cannot find or correctly identify all issues that are root causes or symptoms of operational problems or disruptions. At the same time, the more traditional ML / AI models are so narrow that they only offer limited value, as they have to constantly be adjusted to any contextual changes (new HCI appliance available, new version of vSphere, etc.). Therefore, today we need a combination of deep learning and domain specific models so that we can have a reliable system that is also able to detect issues that were not on the operators’ radars.
Example: Enterprises do not like to buy and operate two types of infrastructure monitoring solutions. Therefore, today’s class of deep learning-focused startups struggle to find their place in the market, as they do not have the ability / resources to ‘inject’ the domain knowledge needed to get to a 99% ‘hit rate’ when it comes to problem detection. Therefore, these startups now often focus on providing higher level business value, for example, to help enterprises optimize resource allocation for their DevOps processes.
Recommendation #5: Do not Get Discouraged by the Absence of Artificial General Intelligence
While Captain Picard’s always context-aware central Startrek computer does not exist today, today’s deep learning neural networks have made tremendous headway, mainly due to much more available infrastructure resources. What should make you specifically optimistic for 2018 is that many 2017 ML / AI projects were stopped due to trivial reasons such as the absence of data streams, indexers, or training UIs that are accessible to business users.
Example: Many of my personal POCs in 2017 failed early because of my inability to quickly and cheaply import data and files. This has a double negative effect, as at the same time this lack of ‘ability to experiment’ prevented me from getting a ‘better feel’ for the potential impact of ML / AI in different scenarios.
Recommendation #6: Implement a Staged Machine Learning and AI Strategy
Your boss and his boss need to know that your ML / AI strategy is routed in reality, but has the vision to capitalize on future technological breakthroughs very quickly. This goes in line with the previous 5 recommendations that all aim to find the optimal compromise between benefitting today, while 'keeping your eyes open' for what's coming down the road. Disappointing today's expectations will make tomorrow's ML / AI projects much harder. Therefore, enterprises require a staged ML / AI strategy that focuses resources where they are most effectively used today, while at the same time taking some risks with ML / AI to already benefit from 'future technologies,' at least to some degree.